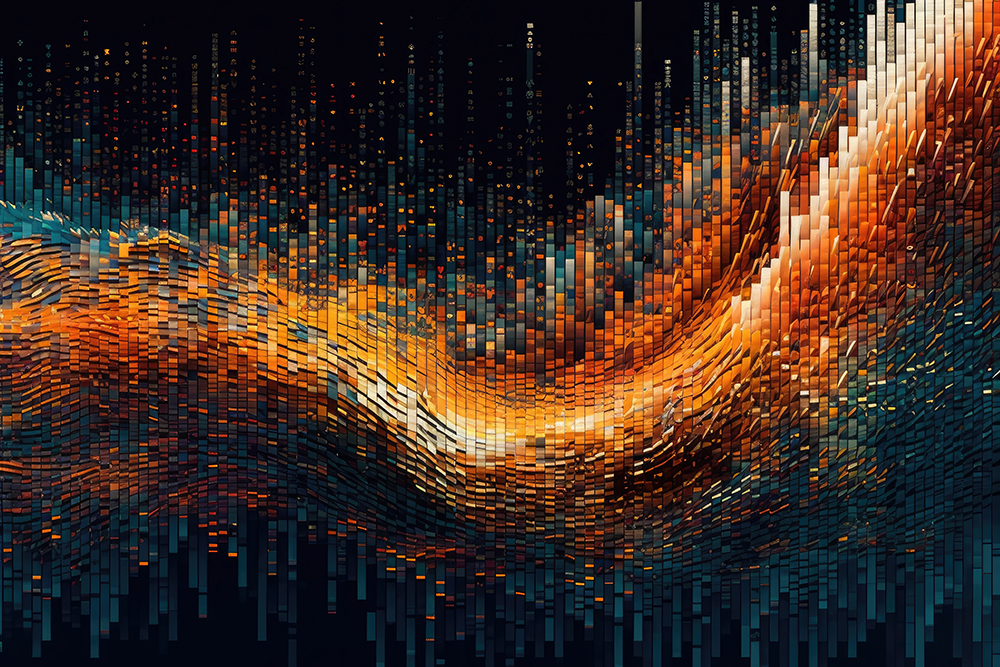
Developing New Medicines Through Artificial Intelligence
On September 30, 2012, a computer program named “AlexNet” won an international competition and ushered in a new age of artificial intelligence (AI). To date, 521 AI tools have received FDA clearance and are improving people's lives worldwide. Over 75% of these tools are targeted at helping radiologists. Curtis P. Langlotz, MD, Ph.D., the current chair of the Radiological Society of North America, predicted that “Radiologists who use AI will replace radiologists who don’t.”
These tools are also gaining acceptance in other areas of healthcare because of a new generation of AI called Large-Language Models (LLM). No doubt you have heard of them in the news, where they have been used as personal assistants that do everything from summarizing doctor visits to writing computer code to creating music.
AI + Protein Folding = New Medicines
LLMs are now being used to develop new medicines and medical treatments. Effectively, biology and chemistry— how we describe life— can be considered languages like English and Mandarin. Instead of nouns and verbs, this language comprises atoms, DNA, genes, and proteins. These and similar models are considered to have solved many aspects of a problem first posited in 1969 by American Biologist Cyrus Levinthal, Ph.D.: How do proteins fold?
Protein folding can be thought of as a kind of molecular Origami. Imagine you are given a piece of paper and a finished origami swan. How difficult would it be to fold that piece of paper into the swan without instructions? How many failed attempts would it take to get it correct? Levinthal suggested that it would take more time than the current age of the universe to determine how a protein should fold, yet living things do this all of the time in fractions of a second.
Proteins are the focus of new medicines that have been successfully used worldwide. For example, antibodies are naturally occurring proteins that help our immune system fight off disease. Scientists can alter the protein sequence of an antibody so that it folds into new shapes that work against new targets. Over 90 such antibodies have been approved by the FDA to treat asthma, cancer, heart disease, rheumatoid arthritis, severe eczema, and infections like SARS-CoV2, the virus that causes COVID. However, developing new antibodies is difficult without understanding how a protein folds into the proper shape. If the shape is not correct, then the antibody won’t work. This is where AI comes in.
Conventional Pharmaceutical Development
Most medicines that are used today were developed over decades of research. Each candidate molecule is a lottery ticket. Pharmaceutical companies screen thousands of potential drug candidates for a reasonable chance that one of those candidates hits the jackpot and can be turned into a medicine (Figure 1). One 2020 study estimated that the average cost to get an effective drug to market between 2009 and 2018 was $1.3 billion. This is one reason that new drugs cost so much.
Figure 1: A conventional pharmaceutical development pipeline takes over a decade to generate a useful, tested, and approved drug.
Identifying a winning drug is even harder for protein-based medicines, such as antibodies. There is more than one correct way to construct a sentence. Similarly, there is more than one way to construct an antibody to fight disease. Each position in the antibody sequence can be made from one of twenty different amino acids. Therefore, the number of possible antibody sequences exceeds the number of molecules in the universe!
It’s challenging (and costly) to perform enough experiments given so many possible sequences. Even if our previous computational methods perfectly predicted if an antibody sequence would work, we might still not have the computational power required to test all these possibilities blindly. This means that the complexity and size of protein-based medicines could further slow the already sluggish conventional drug development pipeline.
Fortunately, AI can help. In 2021, an LLM could predict “protein structures to near experimental accuracy in a majority of cases.” Past computational methods used supercomputers to predict protein folding on one candidate at a time. Several researchers have successfully made these predictions on several million candidates using AI in just a few weeks.
This presents a new solution to the needle-in-a-haystack problem of drug development. Instead of performing costly and time-consuming laboratory experiments to test candidates one by one, AI can learn what makes a suitable antibody and then design thousands of new sequences likely to have these characteristics. In other words, AI can find the right area of the haystack to look at. This will lead to antibody-based medicines that are more effective, cause fewer side effects, require fewer doses, work for more extended periods, and have longer shelf lives. All at a lower cost.
There’s still a problem, though. An AI is only as good as the size and quality of its training dataset. At its core, AI teaches the computer based on patterns in data from previous real-world experiments. AI needs many examples of something to learn how to make a new one. Chatbots get to mine the entire internet to learn how to respond to your question. But how do you train an AI to make your antibody better? You need a way to quickly generate large datasets of natural antibodies and test the ones with the highest chances of winning.
AI + Protein Folding + Cell-free = The Resilience Advantage
Figure 2: Resilience’s cell-free protein synthesis platform offers flexibility, speed, and throughput advantages for drug manufacturing and development. Our cell-free development platform accelerates the use of AI to design new medicines by quickly supplying large protein datasets to inform computational models better.
At Resilience, we combine AI design with large datasets generated using our proprietary, cell-free protein synthesis technology (Figure 2). This allows us to produce and evaluate more antibodies than is possible with traditional cellular methods.
Cell-free protein synthesis uses the biochemical machinery of life outside the confines of a living cell to simultaneously produce and then evaluate thousands of unique candidates from chemically synthesized DNA. Cell-free bypasses previous bottlenecks like cloning, cell transformation, and cell growth, testing more candidates in a day than most systems can in weeks to months.
Furthermore, upon identifying a potential therapeutic candidate, we have efficient processes to characterize and scale for likely therapeutics that can be manufactured (aka developability). This allows new medicines to reach patients faster. We can use this pipeline to optimize or even save promising drug candidates but could use some fine-tuning to make them able to be produced successfully and cost-effectively (Figure 3).
Figure 3: Resilience has established differentiated molecular development capabilities in cell-free and AI that work together with our protein characterization and manufacturing pipelines to accelerate the path to a new drug from multiple starting points in development.
A key advantage of our approach is that it improves continuously and quickly over time. The more data an AI model has, the more accurate it becomes (Figure 4). The more precise the AI model becomes, the more likely it is to find breakthrough new drugs. With cell-free production and evaluation cycles taking just a day or two, we work synergistically with AI to converge on solutions to some of medicine's most challenging problems.
Figure 4: Resilience’s virtuous cycle of AI-driven cell-free protein design continually improves with use. This makes us better and better at turning drug targets from new biological discoveries into new medicines.
A Bright Future for Drug Discovery
Figure 5: Submissions to the FDA have significantly increased in the last few years. (Source: Liu et al.)
The FDA has led in developing best practices for using AI in the drug development process. In 2016, only one FDA submission used AI in the drug development process. There were over 100 submissions in 2021 alone. The tidal wave is upon us.
The incredible acceleration of AI’s integration into drug development cannot be overstated. We are amid the most significant advancements in medicine in decades. Future healthcare professionals may look back incredulously to the time before AI when new treatments were selected with the same probability as winning lottery tickets.
About the Author:
Tony Reina - Principal Data Scientist
Tony Reina is a Principal Data Scientist at Resilience. He is a technical expert in the Advanced Research & Development group, focusing on the areas of protein engineering and cell therapy manufacturing.
Prior to Resilience, Tony was the Chief AI Architect for Health & Life Sciences at Intel for four years At Intel he helped medical device manufacturers incorporate and accelerate AI software into their products.
Tony has an M.D. from the University of Maryland and a Masters in Data Science and Engineering from the University of California San Diego. His post-doctoral work in neuroscience focused on brain-computer interfaces to restore arm movement in paralyzed patients.